By Jonathan Stokes (left), Bruce Guthrie (center), Stewart W. Mercer (right), Nigel Rice, Matt Sutton
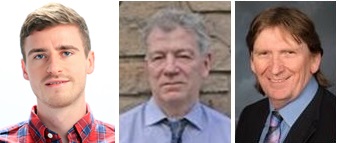
“The problem of multimorbidity,” both for individual patients and for health systems, has been well defined. Multimorbidity is now a well-established priority for research and medical practice [1, 2]. But, there has been little success to date in developing effective or cost-effective new models of care for these patients [3].
Multimorbidity is very different from traditional single disease intervention, however. For multimorbidity, there is huge heterogeneity with many possible combinations of conditions potentially requiring different approaches to management. For example, there are over 268 million possible unique combinations if considering 28 individual conditions. Researchers have therefore begun to search for more useful approaches to dealing with multimorbidity, beyond a count of accumulated conditions, but still simplified to a manageable handful of subgroupings, a focus on “clusters”. The hope is this approach might (i) identify target clusters for direct intervention, or, (ii) via further research on aetiological mechanisms, target clusters for preventing disease accumulation.
Two statistical approaches, (1) cluster analysis (grouping diseases), and (2) latent factor analysis (grouping patients), are commonly used to examine clusters in the general population to accomplish this stratification [4]. However, both approaches have inherent methodological and clinical/intervention complexity. To summarise, on a practical level, they risk producing results that are too abstract (e.g. unobservable latent variables) and generally over-simplifying (e.g. to a handful of combinations when there are actually a huge number that matter) compared to what can actually be observed and acted on in clinical practice: symptoms, signs, and conditions. It is also not obvious that highly prevalent clusters in the general population will be the same combinations associated with outcomes that place most pressure on the supply constraints of healthcare systems, such as costs of (potentially preventable) emergency admissions and overall costs of secondary care.
We drew instead on more simple descriptive analysis to assess all observable condition combinations and their (potentially preventable) secondary care costs [5]. We examined the distribution and top 10 unique multimorbidity combinations contributing to total secondary care costs for a cohort of patients, all (over 8 million) patients with an NHS inpatient admission in England in 2017/18. As well as contribution to total system costs, we examined the combinations with particularly high costs for individual patients. Finally, multimorbidity is dynamic and conditions can accumulate over time, so we looked at the sum of costs for all overlapping conditions in the top 10 (e.g., summing costs for all unique combinations containing, at least, diabetes + hypertension), which might offer priority targets for prevention of disease accumulation.
The main limitations were a focus on a single outcome (costs), in a single healthcare setting (secondary care), with potential under-recording of conditions. However, conditions appeared to be well-recorded and we attempted to backfill missing, healthcare costs are an extremely important outcome for policymakers, and secondary care is the highest cost healthcare setting.
Key findings/implications for policy and practice:
• There are no clear discrete disease combinations at which to target interventions, which implies a generalist/multidisciplinary team approach will remain important rather than pathways/guidelines based on a few specific disease clusters.
• Combinations containing the highest cost patients (the current focus of many interventions) were different to those accounting for the highest total costs, implying the need to develop interventions beyond only high-risk patients.
• There might be scope to use clusters to understand and develop preventative interventions, but focusing on addressing well-known disease risk factors (such as obesity, diet, exercise, and deprivation) with public health/primary care interventions might provide the most efficient route to benefit systems financially and benefit many patients with multimorbidities.
These findings also have implications for researchers/research funders, a need to re-examine how much emphasis is placed on research exploring clusters of multimorbidity, and for which specified reasons.
The article can be assessed at the following link: https://journals.plos.org/plosmedicine/article?id=10.1371/journal.pmed.1003514
References
- Academy of Medical Sciences. Multimorbidity: a priority for global health research. 2018.
- Whitty CJM, MacEwen C, Goddard A, Alderson D, Marshall M, Calderwood C, et al. Rising to the challenge of multimorbidity. BMJ. 2020;368:l6964. doi: 10.1136/bmj.l6964.
- Smith SM, Soubhi H, Fortin M, Hudon C, O’Dowd T. Interventions for improving outcomes in patients with multimorbidity in primary care and community settings. Cochrane Database Syst Rev. 2016;4.
- Ng SK, Tawiah R, Sawyer M, Scuffham P. Patterns of multimorbid health conditions: a systematic review of analytical methods and comparison analysis. Int J Epidemiol. 2018;47(5):1687-704. Epub 2018/07/18. doi: 10.1093/ije/dyy134. PubMed PMID: 30016472.
- Stokes J, Guthrie B, Mercer SW, Rice N, Sutton M. Multimorbidity combinations, costs of hospital care and potentially preventable emergency admissions in England: A cohort study. PLOS Medicine. 2021;18(1):e1003514. doi: 10.1371/journal.pmed.1003514.